

Dragging multiple tiles should no longer lock up the program.
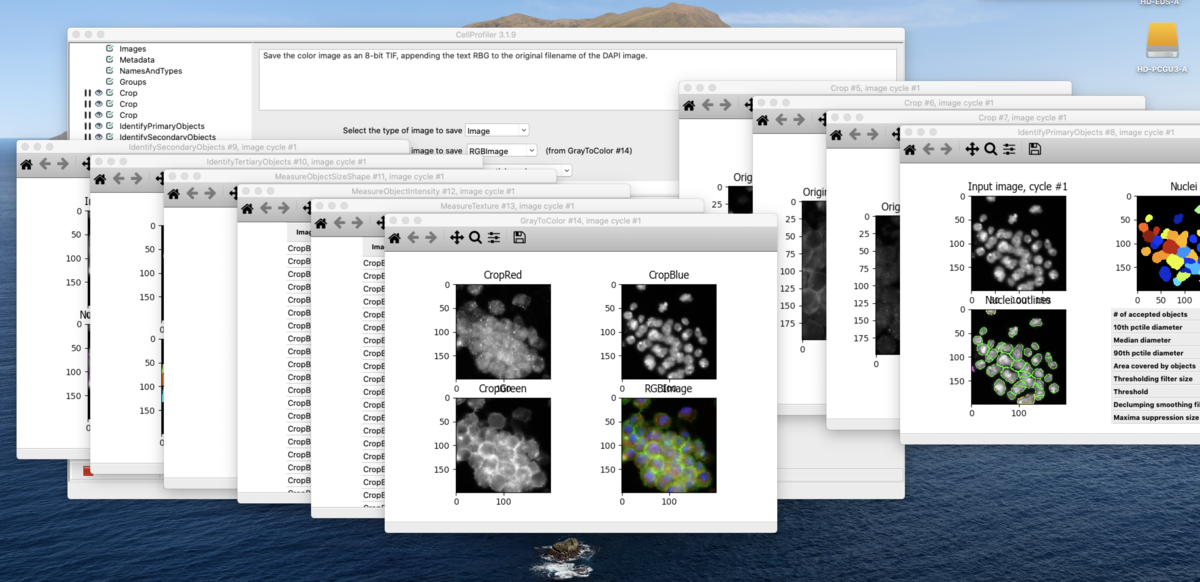
Filters can now operate at the per-object level, rather than being limited to operating on whole images.Scaling is enabled by default on model types which most benefit from it (SVC, KNeighbours and Neural Network). Classifier models now support scaling to normalise data before classification this can be toggled on/off in the “advanced” menu.These can be particularly useful for performing complex non-linear classification tasks. You can now classify objects using customisable neural networks. If a classifier window is open, you can send the selected objects directly to the classifier. Draw around objects to select them, then right click to see options for displaying them. The dimensionality reduction plots have a “ lasso” tool for selecting objects.The available reduction methods include PCA, SVD, Factor Analysis, t-SNE, and several others. This can be useful for identifying outliers and other groups of interest within your data. This plot interface allows you to condense high-dimensional data sets (those featuring many measurements) into a smaller set of components reflecting overall variance. Added the “ Dimensionality Reduction” tool.We’ve added some exciting new features to CellProfiler Analyst:
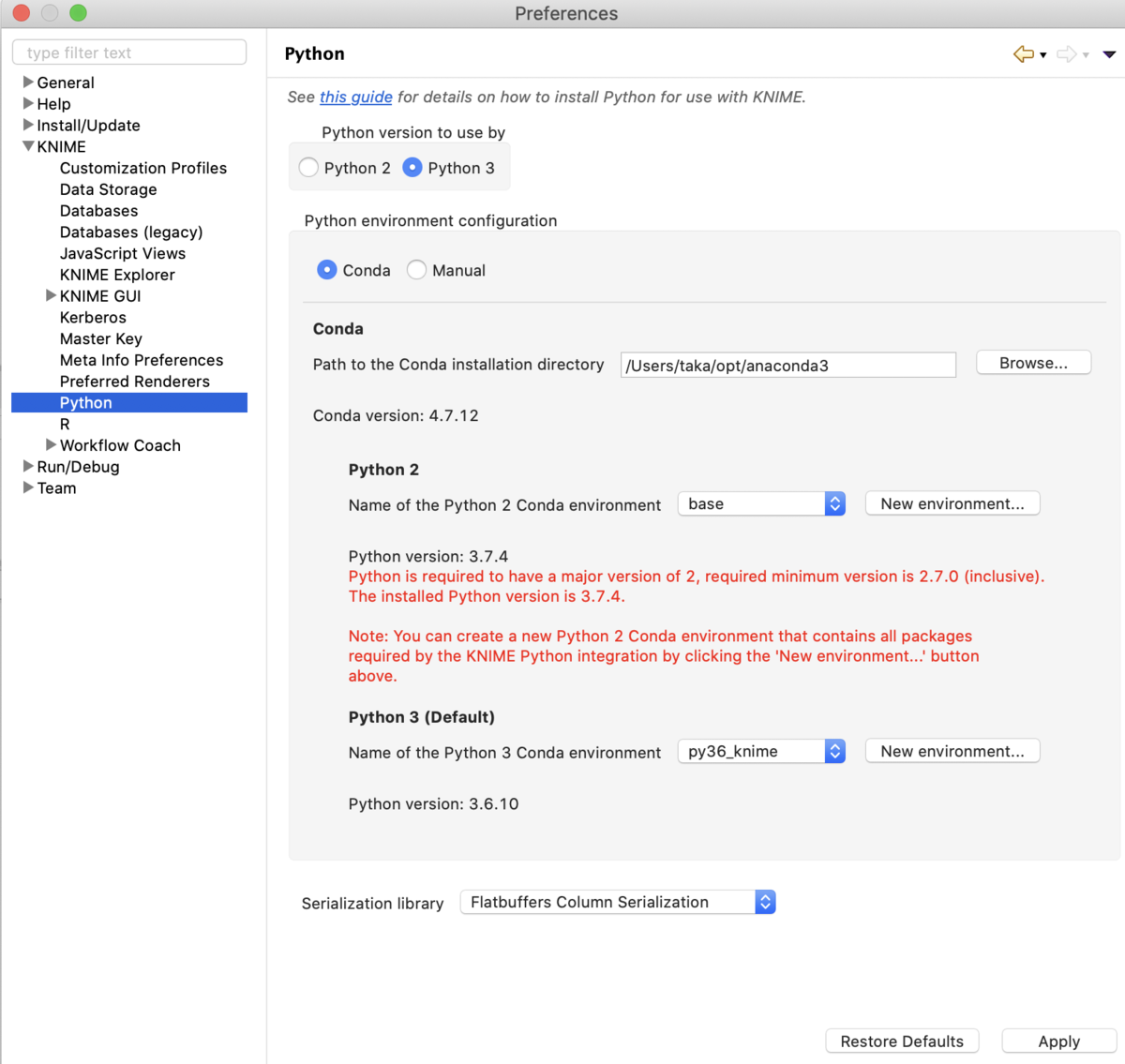
#CELLPROFILER PYTHON SOFTWARE#
Along with backend improvements that make the software faster and more reliable (see Performance), we’ve made some significant changes to how you can easily and efficiently interact with the software (see Interface Refinements) and your data (see New Functionality). We’ve also focused on improving performance and usability.
#CELLPROFILER PYTHON DOWNLOAD#
We have now released CellProfiler Analyst version 3.0! Download it here.Īs with CellProfiler 4, the primary goal of this release has been to migrate from Python 2 to Python 3 and modernise the application.
